Businesses are well aware that analytics are changing everything—and that the difference between simply surviving, or thriving, hinges in how they interpret and utilize data. Through analytics, organizations gain insights that drive decision making about everything from marketing and customer support to accelerating product innovation. However, often, the data that holds the greatest business value, unstructured data, is going untapped.
Unstructured data comes from many channels and sources, both conventional and emerging. Conventional data sources often include: survey data, web browsing data, product purchase data and focus groups. Emerging sources can include: social media data, connected devices/wearables, mobile data, customer service/customer experience data.
Combining the information from all of these sources gives a company a critical view into how customers perceive its brand and how the business is stacking up against the competition. In fact, applying a custom analytics model to unstructured data, literally millions of data points, can reveal deep insight into what drives customers’ purchasing decisions. The average customer commonly moves through a process that’s described as the “purchase decision funnel.”
There are six stages in this funnel, broken down as follows:
Upper funnel:
- Brand awareness: share of voice (SOV) compared to competitors
- Brand perception: how closely a brand is associated with key features for an offering (i.e., performance, reliability, cost, etc.)
- Digital engagement: how effectively a brand engages with potential customers across the digital channels of web, app, social media & YouTube.
Lower funnel:
- Brand consideration: how frequently is a brand present in multiple brand conversations
- Buying experience: how satisfied is the customer at the time of purchase versus competitor brands
- Owner engagement: post-purchase customer advocacy
Intelligence gathered from unstructured data analytics can help brands adapt their business to address gaps in these critical areas. For instance, a brand can invest resources into improving its online SOV to influence consumers at the upper part of the decision funnel, or it can invest more in the customer experience process after a purchase to create greater loyalty and drive engagement at the lower end.
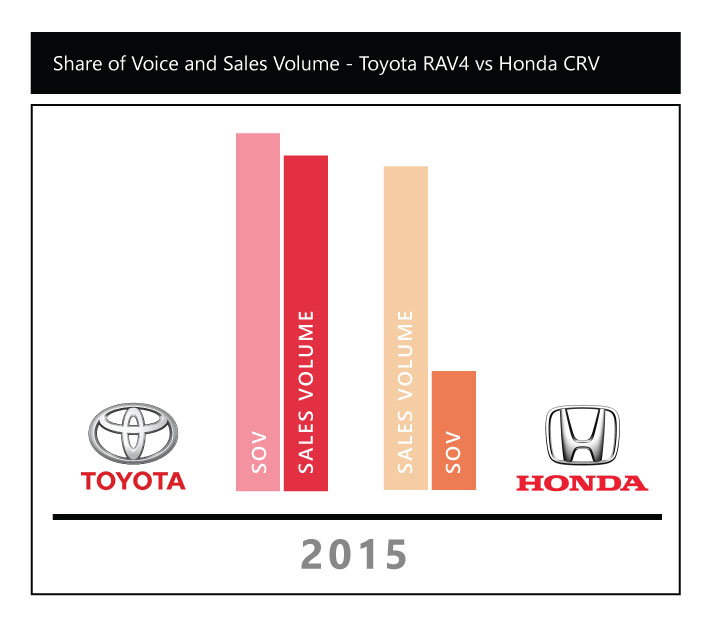
The influence that higher SOV can bring to a brand is at times a double edged sword.
Consider the case of Toyota & Honda compact SUVs (Rav4 & CRV), where Toyota RAV4 had a three times higher SOV compared to Honda CRV in 2015, yet were roughly equal in terms of a sales volume. Toyota RAV4 was plagued by the seat belt recall issue which dominated the social conversations and potentially impacted the customer purchase decisions.
Similarly, Mazda is popular for its comfort and design among consumers and is very also fuel efficient as per internal tests data; however, consumer perception does not reflect this. Mazda’s service sentiment is also low and service levels has been on decline between 2010 to 2015. So possible campaigns need to focus on this message. Improving this could further enhance the number of Mazda brand advocates.
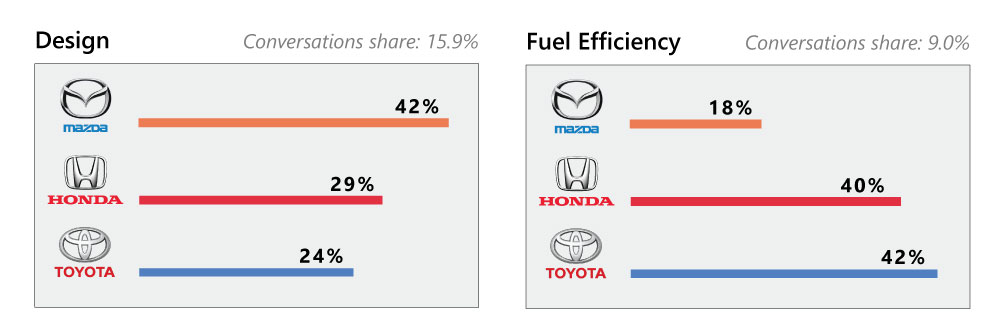
In the one year SOV comparison between Honda CRV, Mazda CX5 & Toyota RAV4, Honda despite having three times lower share of voice than Toyota RAV4 exceeded the sales possibly due to the positivity in the conversations. Hence, for the upper funnel it was observed that Honda has strong associations with key features like performance and comfort and Mazda has weak associations in the consumer’s mind with different features. It was also observed that Mazda is lagging in the digital consumer experience.
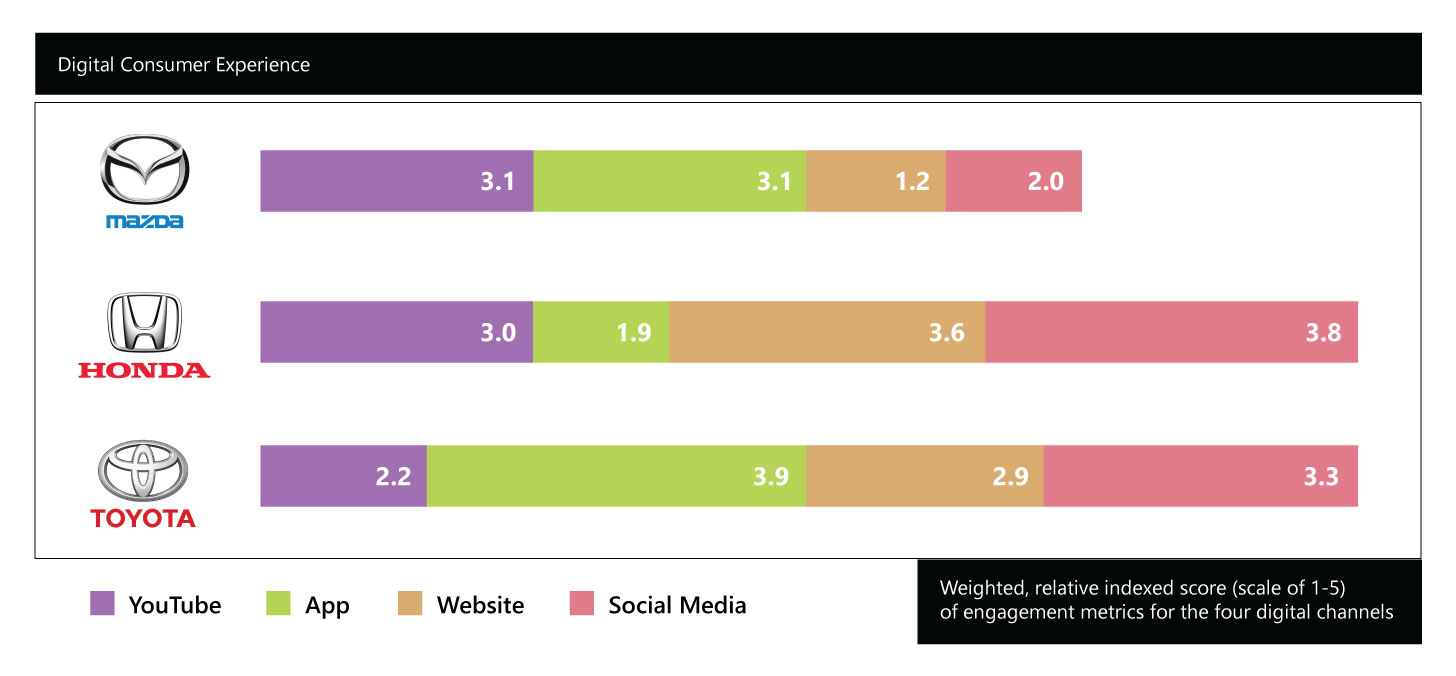
For the lower funnel it was observed that Mazda appears less frequently in the brand consideration set. While Mazda was rated high when it came to sales satisfaction, it scored low in prospect satisfaction suggesting potential lapses in prospective customer treatment at the dealerships. Now that the indices can be obtained at a dealership level, the data could also be analyzed further to identify dealerships that need improvement and dealerships that are performing well; this will enable cross learning opportunities. Further, specific measures like product genius as adopted by Apple retail can be put to test in select dealerships and the improvement can be monitored using these indices.
The decision funnel can reveal insights into the brand perception analysis to help senior management compare expected and actual perception for their brands and fine tune messaging to enhance and modify perception as needed. Consider the case of streaming services player Netflix, considered a giant in media content. Netflix had recently lost some of its lead in shows to online streaming competitor Hulu. Prompt awareness of this change in perception using analytics to understand the change in perception would allow Netflix to make strategic decisions to rechart consumer perceptions about their shows and develop marketing campaigns or content appropriately. The analysis will also serve to continuously monitor the success of these strategic moves.
Take another example; in the case of Mazda, the service sentiment is low and service levels has been on decline between 2010 to 2015. Improving this could further enhance the number of Mazda brand advocates. Further Nissan has shown significant improvement in overall customer retention after it gained a set of loyal customers for it’s niche electric car – Nissan Leaf aided by the IHS loyalty automotive awards. The decision funnel can reveal where and why, what is lacking in your brand.
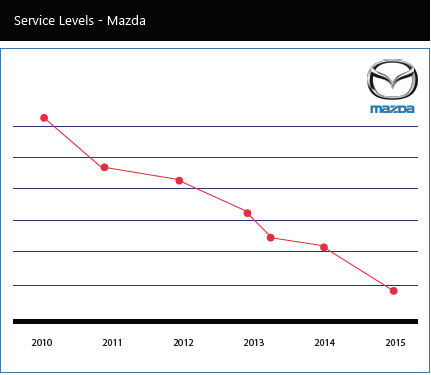
If you are seeking to strengthen your brand, gain competitive advantage and boost sales and revenues, analyzing unstructured data to better understand and impact the decision funnel may be the perfect foundation for this transformation.
No comments:
Post a Comment